Neuroscience
Learning the affordances for maximum distance throwing
Over the last couple of posts, I have reviewed data that shows people can perceive which object they can, in fact, throw the farthest ahead of time by hefting the object. Both the size and the weight of the object affect people's judgements and the distance thrown; however, only weight affects the dynamics of throwing (release angle and velocity are unaffected by changes in size). This rules out the smart perceptual mechanism proposed by Bingham et al (1989), which proposed that both size and weight changes affect hefting and throwing the same way. So how are people perceiving this affordance? What does the affordance space look like? Zhu & Bingham (2010) investigated two options; smart mechanism and function learning.
First, they took advantage of the fact that their previous work (Zhu & Bingham, 2008) had measured the shape of the affordance property to be learned. Figure 1 is from that paper, and shows how distance travelled varies as you vary size and weight (for actual throws; this needs some simulation work too). This function is the affordance and is what people need to learn if they want to be able to perceive the affordance for any given size/weight combination. 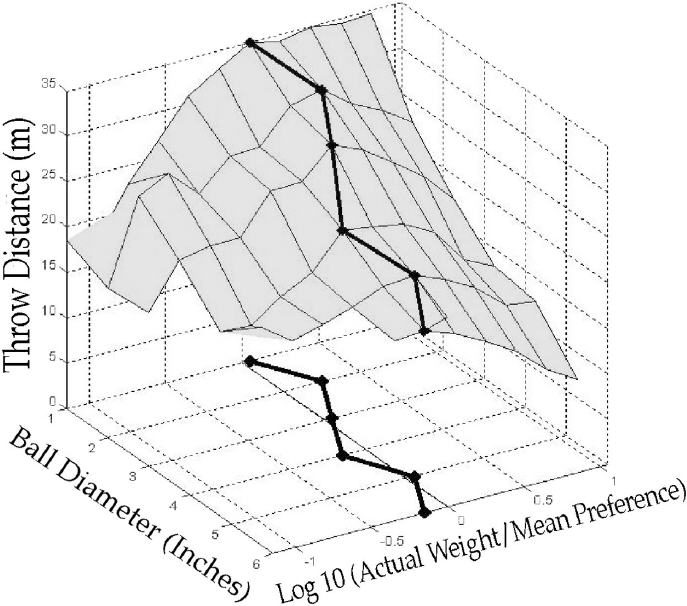 |
Figure 1. Distance thrown as a function of the ball's size and weight (Zhu & Bingham, 2008) |
There are two basic ways to come to 'know' the shape of this function.
First. there might a smart mechanism at work (just not the one Bingham et al suggested). If there is a single variable whose value specifies the distance thrown for any given combination of size and weight, and if this variable is created by both hefting and throwing, a smart mechanism could latch onto this variable during learning.
The second option is function learning, a more common cognitive approach. in which people perceive size, weight and distance thrown (i.e. knowledge of results) separately and learn the function that relates them together. The idea is that each time you throw, you are sampling from this function. You get a size, a weight, and a distance for that size-weight combination. Over time you experience a variety of sizes and weights and resulting distances, and you extrapolate the function that relates them (like regression analysis; it's curve fitting to data). Then, when you're given a novel size-weight combination, you look up this function and generate an estimate for the distance it will travel, allowing you to pick the object that will go the farthest. (If you're familiar with the motor control literature, this is how Richard Schmidt proposes we form schemas and generalised motor programmes).
Figure 1, however, tells us that in this case, the function to be learned is actually quite complicated. Size and weight make independent contributions to distance thrown, so you would need an extensive sample across a wide variety in order to learn the function. A smart mechanism, however, would only need to sample the space in a manner that produced enough variation in distance to be detected and guide the process of differentiating the relevant information variable. The two accounts therefore make different predictions about the consequences of training with limited sets of objects, and Zhu & Bingham (2010) therefore tested the two ideas by training people with different object sets.The Experiment
Everyone (4 groups of 8 novice throwers; all except one happened to be female, but this has more to do with the fact that girls don't get encouraged to throw a lot) were asked to do the hefting task and select the objects they thought they could throw the farthest. They then threw all the objects. They were then trained in one of four experimental conditions: - Constant size (7.62cm diameter)
- Constant weight (69g)
- Constant density (size and weight varied to preserve a density of 0.3g/cm3)
- Constant density with vision of the throw obscured during training (no knowledge of results)
Training was extensive and took place over a month; they were then retested on their throwing and affordance perception using all the balls.
The predictions were - Function learning: each group would only be able to accurately judge throwability to a maximum distance within their training set. So the Constant Size group would have learned the function relating that size with variations in weight to distance, and so would only be able to generalise within that size range because the function changes for different sizes. Similar for the Constant Weight and Density groups.
- Smart mechanism: each group would have experienced enough variation in resulting distance to be able to attune to the relevant information variable that specifies the affordance property mapped in Figure 1. They would therefore be able to perceive the affordance accurately throughout the space (e.g. the Constant Size group would be able to do it even for objects of different sizes).
- If visual information about distance travelled is required to learn the affordance, the final group will not improve at the hefting task even if their throwing improves.
The results were straightforward:- Prior to training, all participants were poor at judging throwability to a maximum distance via hefting. They were also poor at throwing ( on average they could muster no more than 9.6m, compared to the typical 29m seen in Zhu & Bingham, 2008).
- During training, all four groups improved their distances to about the same extent. There was variation in the overall averages, but different groups threw balls of different average weights which affects distance. When Zhu & Bingham compared performance on the ball weights the four groups threw in common, performance was statistically identical.
- After training, all three groups that had visual feedback about their thrown distances during training could perceive the affordance for novel objects via hefting. Their judgments became more reliable and mapped more closely to the balls they actually threw the farthest in post-test. The Constant Density group with No Vision remained unable to reliably judge throwability to a maximum distance, despite equivalent improvements in throwing ability. They only began to improve at the very end, when they repeated the hefting task after having thrown all the balls with vision, but they remained more variable than the other Constant Density group.
To summarise; before training, all four groups threw poorly and could not perceive the affordance via hefting. After training, all four groups improved their throwing ability (roughly doubling their distances) but only the three groups who had visual feedback about their throws during training also learned to perceive the affordance.
This is clearly evidence for a smart mechanism; none of the groups were provided with enough variation across the whole space to learn the entire affordance via function learning. The three vision groups have clearly learned to perceive a variable that works throughout the space, which enables their learning to 'transfer' to untrained size/weight combinations. The no-vision group did not learn the affordance by post-training. However, after they had seen the results of their throws at post-training, their hefting performance did improve rapidly. This fast improvement suggests that they had, in fact, learned to differentiate the relevant information; this perceptual learning requires calibration, however. Even when you have detected a value of the information variable, you still have to place this value on an action relevant scale; calibration is the process of scaling a perceived variable to the relevant action.
So what is this variable that is detected by a smart mechanism?As yet we do not know; however, it seems to relate to felt heaviness, which is a function of size and weight. Felt heaviness exhibits the size-weight illusion (to feel equally heavy, two objects of difference sizes must be different weights) and the size-weight illusion maps very tightly onto judgments of throwability for maximum distance (Zhu & Bingham, 2011). The obvious dynamic property driving felt heaviness was the inertia tensor (dynamic touch) but recent work has ruled this out in the case of the spherical objects used for the throwing studies (Zhu et al, 2012).References
Zhu, Q., & Bingham, G. (2010). Learning to perceive the affordance for long-distance throwing: Smart mechanism or function learning? Journal of Experimental Psychology: Human Perception and Performance, 36 (4), 862-875 DOI: 10.1037/a0018738 Download
-
Hefting For A Maximum Distance Throw
From the task dynamic analysis of throwing for maximum distance, we've identified the fact that for a given release angle and maximum release velocity, there is an object whose size and weight optimises the distance it will travel when thrown. Can...
-
The Task Dynamics Of Throwing To A Maximum Distance
In my last post I went over the formal concept of task dynamics as a way of analysing a task to identify the affordances in that task. This post will examine the task dynamics of projectile motion and relate these to throwing to a maximum distance.This...
-
How Do We Perceive Which Objects Afford Throwing The Farthest?
Previous work has established that people with throwing experience can perceive the affordance of 'throwability'. If you let these people heft objects with a range of sizes and weights, they will confidently select the one they think they can...
-
Perceiving Long Distances In Action Scaled Units
I have so many things I need to write up just now, but it's been a struggle finding the time. I hope to post on Chemero's last chapter, task-specific devices, calibration and some new coordination data soon. In the meantime, I thought I'd...
-
The Size-weight Illusion Is Functional, And It's About Throwing
My colleagues, Geoff Bingham and Qin Zhu, have recently published some fascinating data which has emerged from their work on the uniquely human skill, long-distance throwing. This is a novel and rich perception-action task which Bingham and Zhu (and recently,...
Neuroscience